The AI SaaS Playbook: Build Your Million-Dollar Subscription Model
- Startup Growth Labs
- Apr 17
- 5 min read
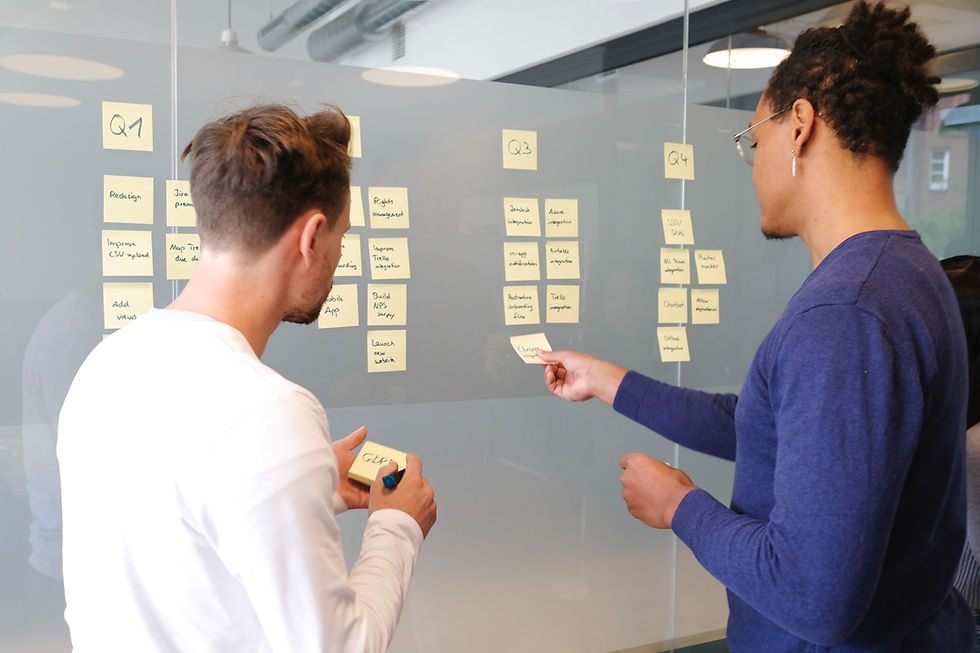
AI SaaS companies are redefining how modern businesses operate—automating repetitive tasks, generating powerful predictive insights, and unlocking efficiencies that were unimaginable just a few years ago.
But here’s the truth: building a successful AI SaaS business isn’t just about the tech. It’s about solving real problems, structuring smart monetization models, and building scalable infrastructure. Without those, even the most advanced AI can fall flat.
Whether you're targeting enterprise buyers or small teams, your product must deliver value with a minimum 30% profit margin to be sustainable. This guide breaks down exactly how to get there—from validating your idea and choosing the right pricing model to scaling your tech and optimizing for retention.
Let’s dive in.
Define Your AI SaaS Product and Market Fit
Success in the AI SaaS landscape begins with solving the right problem. Before writing a single line of code, you need to define your product and find genuine market fit. According to research, 42% of startups fail because they don't address real market needs. So how do you make sure your AI SaaS product doesn't become another statistic?
Identify a real-world problem AI can solve
The foundation of every successful AI SaaS product is identifying a genuine problem that artificial intelligence can solve better than existing solutions. Don't start with the technology—start with the pain points.
What problems should you look for? Focus on these three areas:
Repetitive, time-consuming tasks that could be automated
Complex data sets requiring analysis beyond human capability
Scenarios where predictive insights would create significant value
Examples:
In healthcare, AI can extract insights from clinical notes to support diagnoses.
In agriculture, AI helps predict drought, optimize watering, and monitor crops via drones.
📌 Tip: Avoid general-purpose tools. The most successful AI products solve specific, high-value problems.
Validate your idea with early users
Once you've identified a promising problem, validation becomes critical. According to studies, 90% of startups fail, with lack of market need being a primary reason. Therefore, gathering evidence that confirms your solution addresses a genuine market demand is essential.
How should you approach validation? Engage directly with potential users through:
In-depth interviews with at least 100-200 customers, focusing on those who actually perform the tasks rather than just decision-makers
Surveys that assess willingness to pay for your proposed solution
Creation of landing pages to test conversion rates for different value propositions
Development of a Minimum Viable Product (MVP) to gather real usage data
Here's a crucial tip: ask potential users not just if they want your solution, but if they would pay for it. Customers will often express interest without true purchasing intent. Additionally, categorize problems into three buckets: work being outsourced, tasks requiring expensive consulting, and repetitive processes within the organization. These areas typically represent the greatest opportunities for AI solutions.
Build the Right AI Foundation
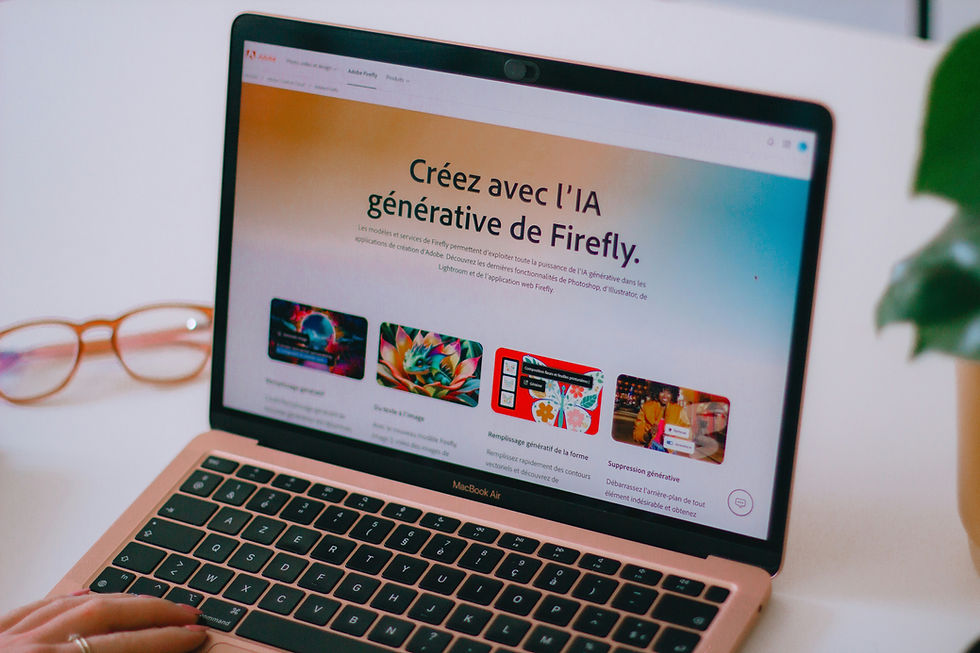
Choose the Right Model for the Job
Match your AI model to the actual task at hand. Most AI capabilities fall into five categories:
Visual Processing (images, videos)
Insight Generation (market intelligence)
Content Creation (text, audio, video)
Speech Recognition
Task Automation
You don’t need to become an AI engineer—your real job is understanding the user’s problem deeply. Then let technical experts handle implementation.
Data Strategy: Quality > Quantity
Great AI starts with great data. Prioritize:
Clean, labeled, structured datasets
High-quality embeddings
Vector databases to support semantic search and LLM memory
Vector databases are essential for features like:
Natural language search
Recommendation engines
Retrieval-Augmented Generation (RAG) to avoid hallucinations
Build Infrastructure That Scales
Choose a cloud provider (AWS, GCP, Azure) based on:
AI tool integration
Global latency and region coverage
Cost structure
Security and compliance
Also:
Use containerization (Docker) for reproducibility
Orchestrate with Kubernetes for dynamic scaling
Track usage with tools like Prometheus and Grafana
Design with auto-scaling and fault tolerance from day one.
Master Your Pricing Strategy
Pick the Right Pricing Model
There are three primary options:
Subscription-based: Best for predictable value delivery. E.g., Microsoft Copilot.
Usage-based (UBP): Charges based on consumption. Ideal for API-based services. E.g., OpenAI.
Hybrid: Combines subscription + UBP. Now used by 46% of SaaS companies.
📌 Hybrid pricing offers flexibility and better aligns value with cost.
Account for AI’s Cost Volatility
AI introduces unpredictable costs from:
Token usage and inference
Model training/fine-tuning
Data processing at scale
This makes usage-based pricing hard to predict. During recessions, UBP sales cycles lengthen 29% vs. just 21% for subscriptions.
Pro tip: Offer free tiers or metered usage for easy adoption, while scaling with usage.
Avoid These Common Pricing Traps
❌ Overcomplicated pricing models
❌ Outcome-based billing (too hard to define "success")
❌ Static pricing that doesn't evolve
❌ Delayed shift to annual contracts
Instead, keep pricing simple. Layer in guardrails like rate limiting and usage dashboards to protect profitability.
Use AI to Build a Smarter Business
Don’t Just Build AI—Use It Internally
Top AI SaaS founders don’t just sell AI—they run their business with it.
Look for tools that:
Automate repetitive workflows
Analyze operations faster than humans
Enhance CX through personalization
Improve dev productivity and QA
Companies that integrate AI internally report up to 34% efficiency gains.
Build a Backbone for Automation
A high-functioning AI-powered company needs:
Unified data access and governance
API integrations between tools
Cloud AI services for compute-heavy tasks
This “AI nervous system” connects your tools and customer touchpoints, enabling intelligence across every department.
Automate Key Operations
Start small: Automate the obvious time-wasters first. Then scale.
Top automation use cases:
Data analysis & reporting
Customer support via AI chat
Marketing personalization
Predictive analytics for retention
Use AI to free your team from grunt work so they can focus on strategy and innovation.
Optimize for Retention, Profitability & Scale
Cut Idle Resources to Save Cash
AI workloads can be expensive—cloud costs alone often eat 6–12% of revenue.
Ways to cut:
Autoscale resources based on usage
Auto-decommission idle instances
Use spot instances where possible (up to 90% cheaper)
Companies like Spotify master this by scaling GPU workloads up/down based on demand.
Bundle Value for Enterprise Clients
Enterprise buyers want packages—not point solutions.
Bundle:
Core AI tools
Data services
Custom integrations
Compliance/security features
Use AI to analyze feature combinations that boost retention. Tailor bundles based on customer segment behavior, not just intuition.
Let Usage Data Guide Your Roadmap
Track:
Feature adoption
Engagement duration
Retention impact per feature
Then use that data to double down on what’s working. Avoid the “gut-feel roadmap” trap—let your users’ behavior lead the way.
Final Thoughts
The path to building a million-dollar AI SaaS business is clear—but it’s not easy. It takes more than brilliant tech. You need discipline in validation, infrastructure, pricing, and scaling.
But with the right playbook, you’re not just building an app. You’re building a business that grows on its own momentum.
👉 For more frameworks, pricing templates, and growth tools tailored to AI startups, visit www.startupgrowthlabs.com
Let’s build something game-changing.
留言